Currently, no cost-effective method exists to efficiently, reliably, and accurately capture analog meter readings in a digital format. This paper details how artificial intelligence was used to capture analog field-gauge data with a dramatic reduction of cost and an increase in reliability. This solution was implemented in the Cheleken oil field in the Caspian Sea offshore Turkmenistan. During the field trial, operators were required to take pictures of the gauges at given intervals and upload the photos to the application.
×
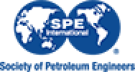
Continue Reading with SPE Membership
SPE Members: Please sign in at the top of the page for access to this member-exclusive content. If you are not a member and you find JPT content valuable, we encourage you to become a part of the SPE member community to gain full access.